Artificial intelligence (AI) technology has made significant strides in recent years, revolutionizing various industries and enhancing the way we interact with digital platforms. One of the most prominent advancements in AI technology is the development of Generative Pre-trained Transformers (GPT), such as GPT-3 and GPT-4 by OpenAI. These models have showcased remarkable capabilities in natural language understanding and generation, making them powerful tools for a wide range of applications. In this article, we will explore GPT with AI technology, examining its pros and cons, how it detects and utilizes data from Google, its ability to provide accurate and authentic information, and the history of AI.
Understanding GPT and AI Technology
What is GPT?
GPT, or Generative Pre-trained Transformer, is a language model created by OpenAI. This model understands and generates human-like text based on the input it receives. By pre-training on a diverse range of internet text, GPT can perform various language tasks, including translation, summarization, question answering, and content creation.
How GPT Works
GPT models operate using a transformer architecture, which relies on attention mechanisms to process and generate text. The key steps in the functioning of GPT are:
- Pre-training: During this phase, the model is exposed to a vast amount of text data from the internet. It learns patterns, grammar, facts, and some reasoning abilities by predicting the next word in a sentence.
- Fine-tuning: In this phase, the model is further refined using specific datasets that are relevant to particular tasks or domains. This enhances its performance in those areas.
- Inference: Once trained, GPT can generate human-like text based on the input provided. It uses its pre-trained knowledge to understand the context and produce coherent and relevant responses.
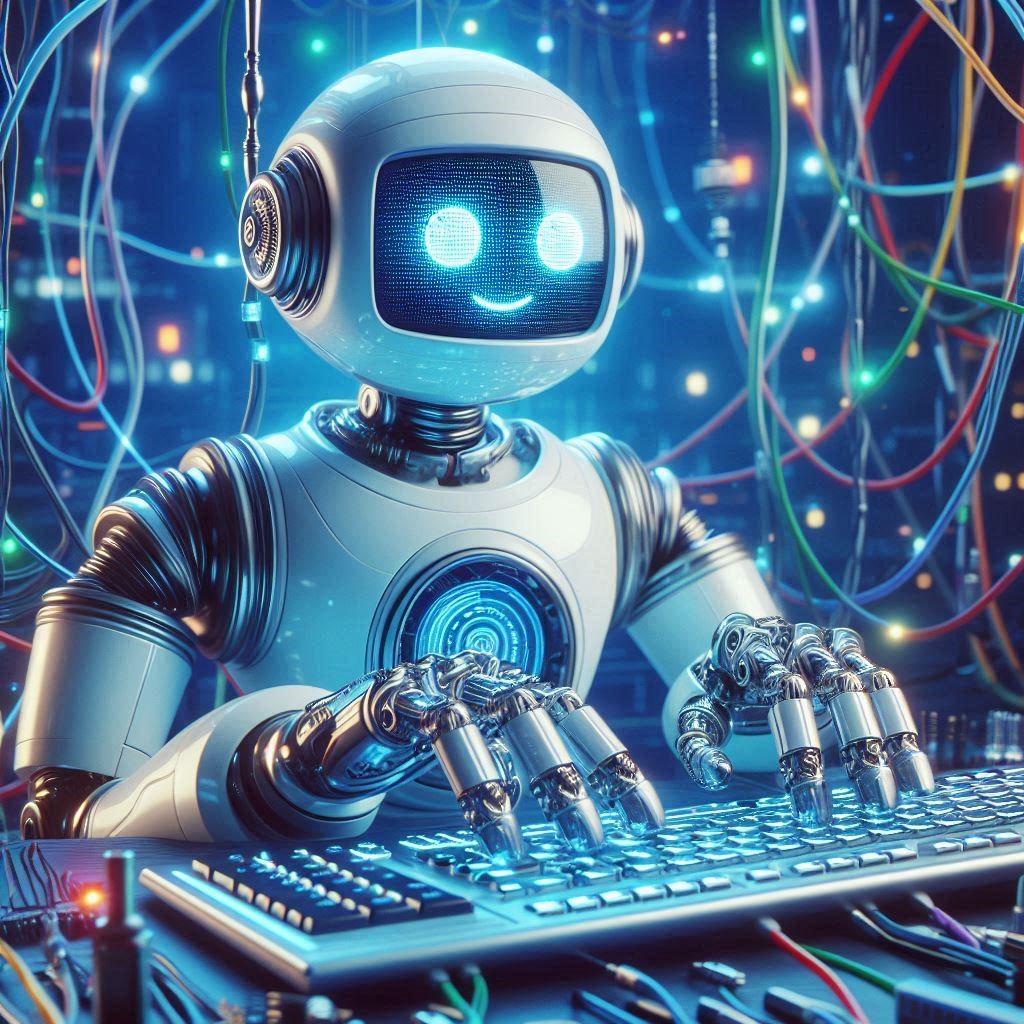
The History of AI:
The history of AI began in the 1950s with foundational work by pioneers like Alan Turing and John McCarthy, who introduced concepts such as machine learning and artificial intelligence. Over the decades, AI evolved from simple rule-based systems to advanced deep learning models, revolutionizing fields such as natural language processing and computer vision.
Early Beginnings
The concept of artificial intelligence dates back to ancient times, with myths and stories about mechanical beings endowed with intelligence. However, the formal study of AI began in the mid-20th century.
1950s: The Birth of AI
- 1950: Alan Turing, a British mathematician and logician, introduced the idea of a machine that could simulate any human intelligence in his paper “Computing Machinery and Intelligence.” He proposed the famous Turing Test to determine whether a machine could exhibit intelligent behavior indistinguishable from that of a human.
- 1956: The term “Artificial Intelligence” was coined during the Dartmouth Conference, organized by John McCarthy, Marvin Minsky, Nathaniel Rochester, and Claude Shannon. This event is considered the birth of AI as a field of study.
1960s-1970s: The Early Years
- During the 1960s and 1970s, researchers developed early AI programs, including rule-based systems and simple problem-solving algorithms. However, progress was slow due to limited computing power and the complexity of AI problems.
1980s: The Rise of Expert Systems
- The 1980s saw the rise of expert systems, which were AI programs designed to emulate the decision-making abilities of human experts. These systems were used in various fields, such as medical diagnosis and financial analysis. Despite their success, expert systems had limitations and required extensive manual knowledge engineering.
1990s-2000s: Machine Learning and Big Data
- The 1990s and 2000s marked a shift from rule-based AI to machine learning, where systems could learn from data and improve over time. This era saw the development of algorithms like decision trees, neural networks, and support vector machines. The advent of big data and increased computing power further accelerated AI research.
2010s: The Deep Learning Revolution
- The 2010s witnessed a breakthrough in AI with the rise of deep learning, a subset of machine learning that uses multi-layered neural networks to model complex patterns in data. This revolution was fueled by advancements in hardware (such as GPUs) and the availability of large datasets.
- 2012: The ImageNet competition showcased the power of deep learning, with deep neural networks achieving unprecedented accuracy in image classification tasks.
2020s: The Era of Transformers
- The 2020s have been characterized by the emergence of transformer-based models, such as GPT-3 and GPT-4. These models have demonstrated remarkable capabilities in natural language processing, generation, and understanding.
-
Image Source: bing.com
History of AI: Key Milestones
Year |
Milestone |
1950 | Alan Turing proposes the Turing Test |
1956 | Dartmouth Conference: Birth of AI |
1960s-70s | Development of early AI programs and rule-based systems |
1980s | Rise of expert systems |
1990s-2000s | Shift to machine learning and big data |
2010s | Deep learning revolution |
2012 | ImageNet competition showcases deep learning |
2020s | Emergence of transformer-based models (GPT-3, GPT-4) |
Pros and Cons of GPT with AI Technology
To better understand the strengths and limitations of GPT with AI technology, let’s examine the pros and cons in the table below:
Pros |
Cons |
High-quality text generation | Potential for biased outputs |
Versatile applications | Resource-intensive training |
Improved natural language understanding | Dependence on training data quality |
Enhanced productivity | Ethical and misuse concerns |
Scalability | Interpretability issues |
Pros
- High-quality text generation: GPT models produce text that is coherent, contextually relevant, and often indistinguishable from human-written content.
- Versatile applications: GPT handles various tasks, such as chatbots, content creation, translation, summarization, and more.
- Improved natural language understanding: GPT enhances human-computer interaction by generating human-like text.
- Enhanced productivity: GPT automates content generation and other language tasks, significantly boosting productivity across multiple fields.
- Scalability: GPT models scale to manage large datasets and complex tasks, making them suitable for numerous applications.
Cons
- Potential for biased outputs: GPT may generate biased or harmful content based on its training data, which might include biased information.
- Resource-intensive training: Training large GPT models demands substantial computational resources and energy, leading to high costs and environmental impact.
- Dependence on training data quality: The quality of GPT’s output heavily relies on the quality of the data used during training.
- Ethical and misuse concerns: GPT can be used maliciously, such as creating fake news, spam, or deepfakes, raising ethical and security issues.
- Interpretability issues: Understanding how GPT models make specific decisions or generate outputs can be challenging, affecting transparency and trust.
How GPT Detects and Utilizes Google Data
GPT models do not directly access Google’s data or databases. Instead, they are pre-trained on a large corpus of text data from the internet, which may include publicly accessible information. Here’s how GPT processes this information:
- Data Pre-training: During pre-training, GPT models learn from a diverse set of internet text, including articles, books, and websites. The model recognizes patterns and language structures by predicting the next word in a sentence.
- Contextual Understanding: When a user provides input, GPT uses its pre-trained knowledge to understand the context and generate responses based on its internal knowledge base.
- Generating Responses: GPT creates responses using the patterns and information learned during pre-training. It does not perform real-time searches or access external databases like Google but relies on accumulated knowledge from pre-training data.
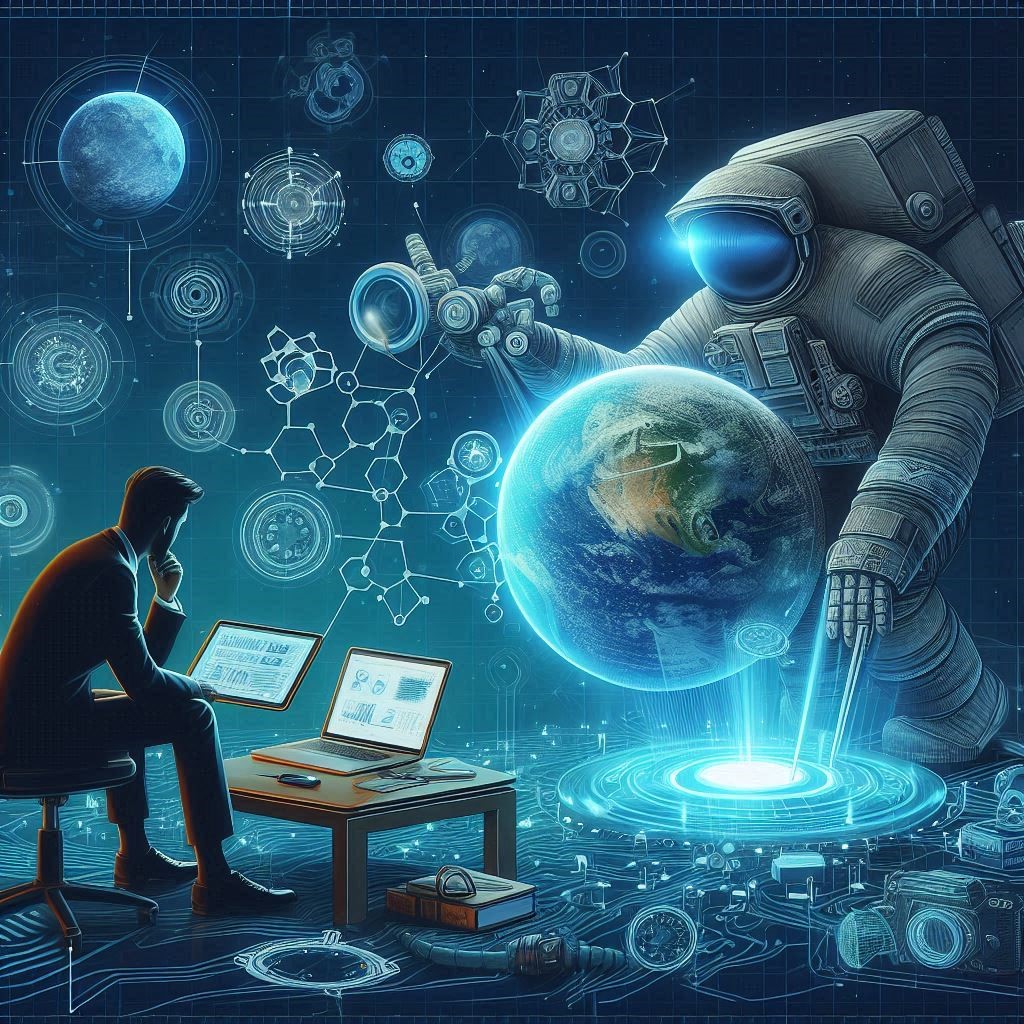
Ensuring Accuracy and Authenticity
To address concerns about AI-generated content, GPT models use the following strategies:
- Fine-tuning with Reliable Data: Fine-tuning GPT with high-quality, verified datasets improves the accuracy and reliability of generated content, minimizing the risk of biased or incorrect outputs.
- Human Review and Oversight: Implementing human review and oversight helps ensure content quality and accuracy. Reviewers verify and correct information before publication.
- Transparency and Source Attribution: Providing transparency about training data sources enhances trust in GPT-generated content. While GPT does not cite sources directly, informing users about the training data’s general nature builds confidence in the information’s reliability.
- Continuous Monitoring and Updates: Regular monitoring and updating of GPT models maintain content accuracy and relevance. Incorporating new, reliable data ensures the model stays current with the latest information.
Conclusion
GPT with AI technology has revolutionized the field of natural language processing and generation, demonstrating remarkable capabilities and applications. From improving human-computer interaction to boosting productivity in various domains, GPT models like GPT-3 and GPT-4 have set new standards for AI performance. However, it is essential to address the challenges and ethical concerns associated with these technologies to ensure their responsible and beneficial use. As AI continues to evolve, the integration of GPT models with other advanced AI tools will further expand the possibilities and impact of artificial intelligence.
FAQs
1. What is GPT? GPT, or Generative Pre-trained Transformer, is a type of language model developed by OpenAI.
2. How does GPT work? GPT models operate using a transformer architecture, which relies on attention mechanisms to process and generate text. The key steps include pre-training on a large dataset, fine-tuning for specific tasks, and generating text based on input.
3. What are the pros and cons of GPT with AI technology? Pros include high-quality text generation, versatile applications, improved natural language understanding, enhanced productivity, and scalability. Cons include the potential for biased outputs, resource-intensive training, dependence on training data quality, ethical and misuse concerns, and interpretability issues.
4. How does GPT detect and utilize Google data? GPT models do not directly access Google’s data. They are pre-trained on a large corpus of text data from the internet, including publicly accessible information. The models generate responses based on this pre-trained knowledge without real-time searches or direct access to Google’s databases.
5. How can the accuracy and authenticity of GPT-generated content be ensured? Ensuring accuracy and authenticity involves fine-tuning with reliable data, implementing human review and oversight, providing transparency about data sources, and continuously monitoring and updating the models.
6. What are some AI tools used for generating images, audio, logos, and websites? There are several AI tools for different applications:
- Image Generation: DALL-E, DeepArt, Artbreeder
- Audio Generation: Jukedeck, AIVA, Amper Music
- Logo Generation: Logojoy, Looka, Tailor Brands
- Website Development: Wix ADI, Bookmark, The Grid
7. What is the history of AI? AI history includes early concepts in ancient times, the formal study in the 1950s with Alan Turing’s work, the rise of expert systems in the 1980s, the shift to machine learning in the 1990s and 2000s, the deep learning revolution in the 2010s, and the emergence of transformer-based models like GPT in the 2020s.
8. How has AI technology evolved over the years? AI technology has evolved from early rule-based systems to machine learning and deep learning, with significant advancements in computing power and data availability.